The 12th IASTED International Conference on
Artificial Intelligence and Applications
AIA 2013
February 11 – 13, 2013
Innsbruck, Austria
KEYNOTE SPEAKER
Uncertainty, Degrees of Truth and Graded Epistemic Logic
Abstract
The aim of the talk is to reports on recent results that bridge the gap between uncertainty representations such as possibilistic logic, modal logic and many-valued logics, especially three and four-valued logics of the unknown and the contradictory. There is a recurrent confusion between degrees of truth and degrees of belief that has created a kind of epistemological mess in the area of knowledge representation. Based on the idea that truth degrees are ontic notions that are a matter of representational conventions, we claim that epistemic notions of knowledge and belief lie at the meta-level with respect to truth-values. We present a minimal epistemic logic (MEL) that can be viewed as either a fragment of a standard modal logic, or as a two-tiered propositional logic of incomplete information. Its semantics can be devised in the setting of possibility theory. We outline the graded extension of this logic, that subsumes possibilistic logic as a special case. We propose several examples of using this setting for knowledge representation tasks;
- Most three-valued logics (lie Lukasiewicz's and Kleene's) where the third truth-value is supposed to encode the idea of "unknown" can be equivalently encoded in a fragment of MEL.
- Answer-set programming (ASP) rules can be encoded in a graded extension of MEL, with three possibility levels.
- The 4-valued truth set of Belnap logic can also be captured by a generalization of MEL The main merit of such a modal encoding of man-valued logic or logic programming formalisms is to lay bare the epistemic content of formulas thus clarifying their meaning, as opposed to the usual simple but semantically opaque syntax of many-valued logics as well as ASP
Biography of the Keynote Speaker
Didier DuboisCo-Editor-in Chief, Fuzzy Sets and Systems (http://www.elsevier.com/locate/fss
)
IRIT, Porte 308.
Postal address
UNIVERSITE PAUL SABATIER
IRIT - (Equipe ADRIA)
(Didier Dubois)
118 route de Narbonne
31062 TOULOUSE CEDEX 9, FRANCE
(http://www.irit.fr)
Tel :+335 61.55.63.31 (05 61.55.63.31 en France)
E-mail : dubois@irit.fr
Fax : +335 61.55.62.39 (05 61.55.62.39 en France)
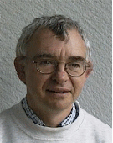
is a Research Advisor at IRIT, the Computer Science Department of Paul Sabatier University in Toulouse, France and belongs to the French National Centre for Scientific Resarch (CNRS). He holds a Doctorate in Engineering from ENSAE, Toulouse (1977), a Doctorat d'Etat from Grenoble University (1983) and an Honorary Doctorate from the Faculté Polytechnique de Mons, Belgium (1997). He is the co- author, with Henri Prade, of two books on fuzzy sets and possibility theory, and more than 15 edited volumes on uncertain reasoning and fuzzy sets. Also with Henri Prade, he coordinated the HANDBOOK of FUZZY SETS series published by Kluwer (7 volumes, 1998-2000, 2 of which he co-edited). It includes the book Fundamentals of Fuzzy Sets, edited again with H. Prade (Kluwer, Boston, 2000). More recently, he co-edited the tutorial handbook Decision-making Process - Concepts and Methods, ISTE London & Wiley, 2009. He has contributed about 200 technical journal papers on uncertainty theories and applications. Didier Dubois
Since January 1, 1999, Didier Dubois has been co-Editor-in -Chief of
Fuzzy Sets and Systems. He is an Advisory Editor of the IEEE
Transactions on Fuzzy Systems. He is a member of the Editorial Board of several technical journals, such as the International Journal on Approximate Reasoning, General Systems, Journal of Applied Logic and Information Sciences among others. He is a former president of the International Fuzzy Systems Association (1995-1997). In 2002 he received the Pioneer Award of the IEEE Neural Network Society, and in
2012 the Scientific Excellence Award from the European Society For Fuzzy Logic And Technology (EUSFLAT).
His topics of interest range from Artificial Intelligence to Operations Research and Decision Sciences, with emphasis on the modelling, representation and processing of imprecise and uncertain information in reasoning, problem-solving tasks and risk analysis.